The Growing Importance of Graph Retrieval Augmented Generation
Combine the power of information retrieval with generative language models to produce responses that map to a knowledge base, are contextually rich, and highly relevant
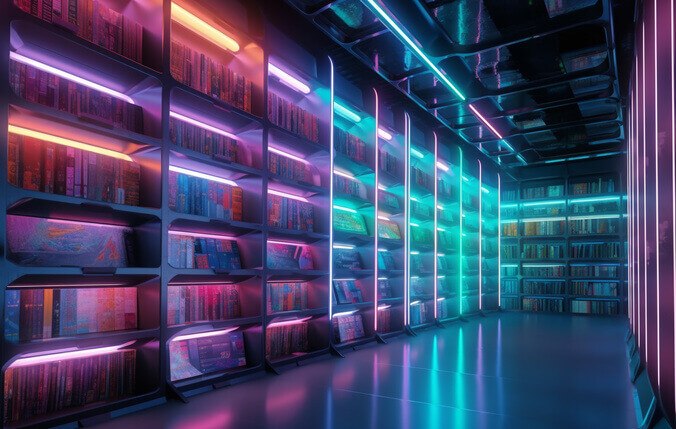
How Baseline RAG Works
Retrieval-Augmented Generation (RAG) operates by first retrieving relevant information from large-scale knowledge sources, such as databases, documents, or the internet. This retrieved information serves as the contextual foundation for subsequent generation tasks. Leveraging this contextual understanding, the generative model then produces responses or generates text that is not only fluent and coherent but also deeply informed by the retrieved knowledge. By combining the strengths of both retrieval and generation, RAG enables our solutions to craft responses that are highly accurate, contextually relevant, and tailored to the specific needs of our clients.
Advantages of Baseline RAG
Contextual Relevance and Factual Accuracy
RAG models excel in producing text that is not merely linguistically sound but also contextually grounded and factually accurate
Enhanced Question Answering Systems
RAG significantly elevates the capabilities of question answering systems by providing them with access to extensive knowledge bases. This facilitates the retrieval of precise information, enabling more accurate and comprehensive answers to user queries.
Improved Summarization and Dialogue Systems
RAG models augment the efficiency and quality of summarization tasks by leveraging external knowledge to produce concise and informative summaries.
Using Graph AI to Improve RAG
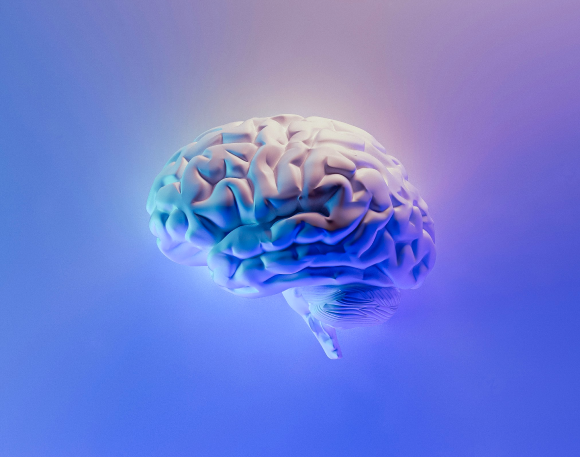
Semantic Understanding through Knowledge Graphs
Knowledge graphs provide a structured representation of information, capturing entities and their relationships in a graph format. In the context of RAG, these graphs can be leveraged to impart a deeper semantic understanding to the retrieval process and enhancing link prediction.
Nodes in the knowledge graph represent entities, and edges denote relationships, allowing for a more fine-grained exploration of interconnected concepts. This enhanced semantic understanding enables the retrieval component to discern subtle nuances, improving the relevance and accuracy of the retrieved information.
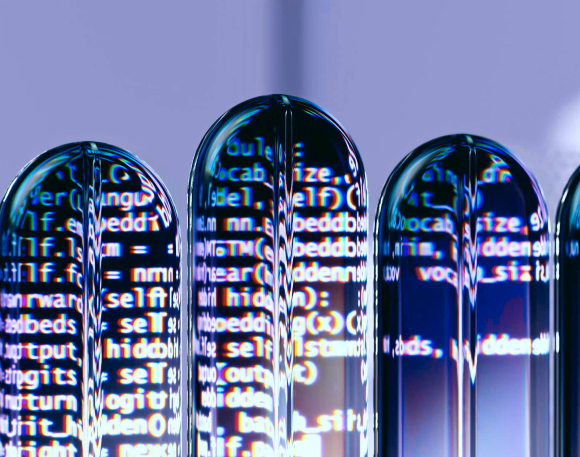
GNNs Powering Graph RAG
Graph AI, particularly Graph Neural Networks (GNNs), excels in learning intricate patterns and relationships within graph-structured data. Applied to knowledge graphs, GNNs can decipher the complex interconnections between entities, uncovering latent associations that may not be immediately apparent.
This capability is invaluable in the retrieval phase of RAG, where understanding the relationships between entities enhances the system's ability to retrieve contextually relevant information. For instance, in a medical knowledge graph, the relationships between symptoms, diseases, and treatments can be harnessed to improve the precision of medical information retrieval and prediction of associated conditions.
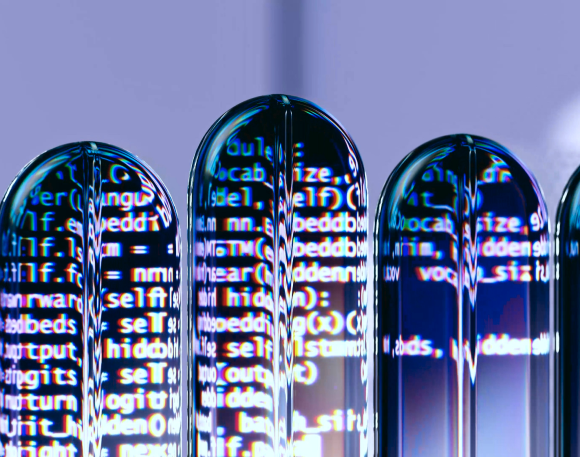
What is Graph RAG
and Why Does It Matter?
The Lynx Analytics Graph RAG Framework
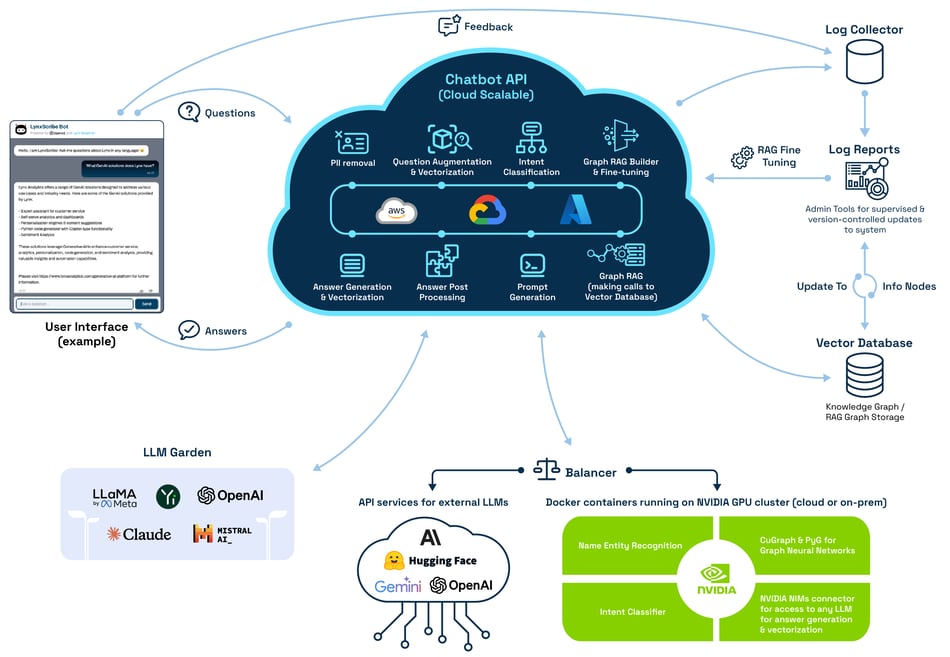
Our Technology Partners
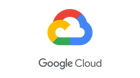
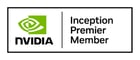
.png?width=140&height=34&name=OpenAI_Logo.svg%20(1).png)
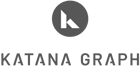
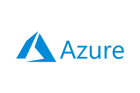
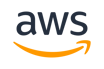
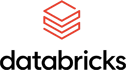
Why Choose Lynx Analytics?
As pioneers in leveraging RAG technology, Lynx offers unparalleled expertise and innovation in harnessing the power of Retrieval-Augmented Generation. With our commitment to delivering contextually rich and highly relevant solutions, we empower businesses to achieve their goals with precision and efficiency. At Lynx, we prioritize accuracy, relevance, and tailor-made solutions, ensuring that our clients receive the highest quality service. Choose Lynx as your trusted partner in navigating the complexities of AI-driven solutions and unlock the full potential of RAG technology for your enterprise.