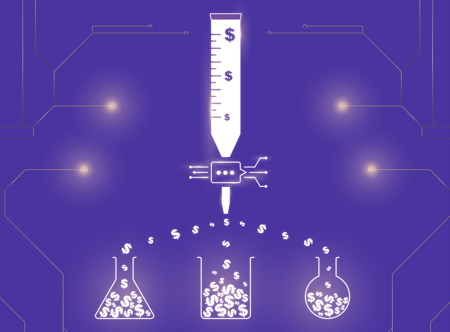
Topics
Select Tab
- All
- Life Sciences Analytics
- Retail Analytics
- Telecom Analytics
- Banking Analytics
- Data Science
Content types
Select Tab
- All
- Blog
- Customer Stories
- E-Books & Guides
- White Papers
Apply Filter
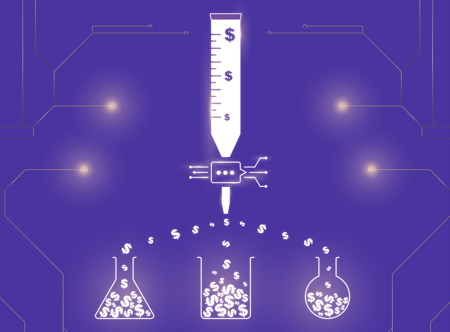
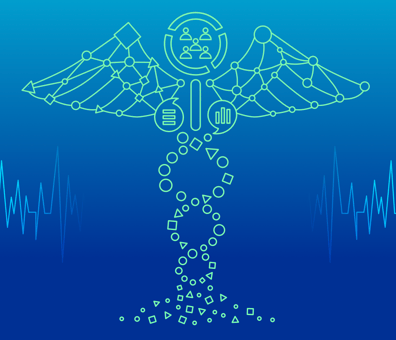
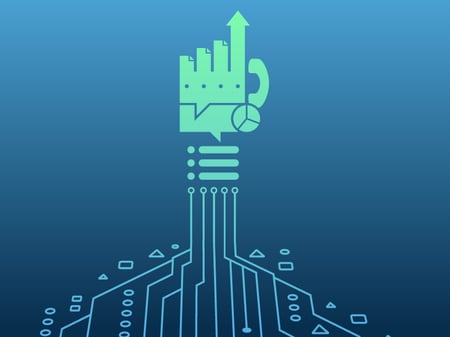
Customer Stories
Fostering Patient Engagement and Trust
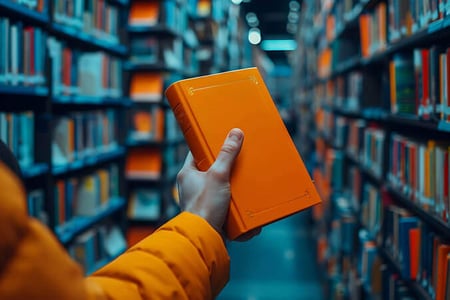
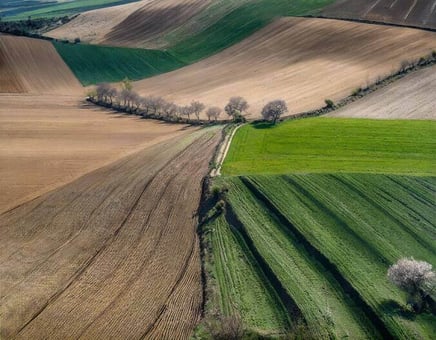
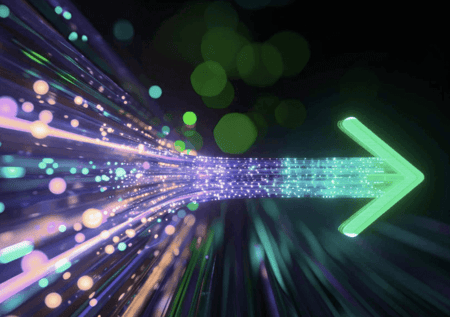
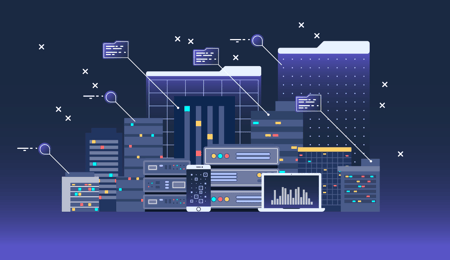
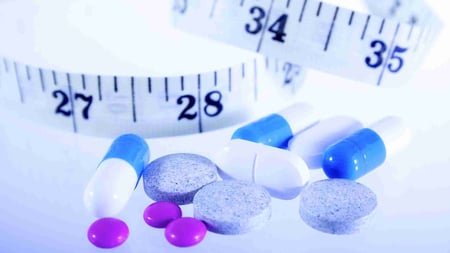
No Result Found
Load More